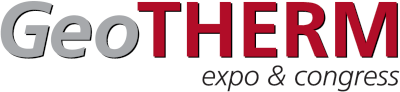
Geothermal energy is a highly reliable, eco-friendly, sustainable, and clean energy source that has proven to be a game-changer in the residential and industrial sectors. It can be developed from hot rocks saturated in geologically favorable reservoirs, in which water is produced at temperatures greater than 120 °C from a depth of up to 4 km utilizing an Electric Submersible Pump (ESP). Once its heat is converted to electricity in the power plant, the water is cooled and reinjected into the reservoir.
Due to the flow rates required, high-enthalpy fluids, and harsh downhole conditions of geothermal wells, a real-time well manager system was implemented to improve the ESP design, operation, reliability, and well performance. This paper details the operating conditions of a high-efficiency geothermal ESP system in Germany with in-house developed machine learning models. Our geothermal ESP well manager system has advanced to obtain virtual measurements, visual operating indices, vibrations tracking, real-time pump and well performance evaluation, electrical unbalance tracking, and scale detection.
The machine learning models predicted pump intake pressure, motor temperature, fluid temperature, flow rate, and overall operating parameters with less than 3% error. Additionally, the virtual parameters and real-time total dynamic head were analyzed together to indicate potential scale buildup within the flow meter, organic deposition on the motor housing, and changes in fluid composition.
A thorough assessment was made by continuously monitoring (24/7) the physical and digital aspects of the system, enabling recommendations to be made for improving power consumption and increasing the lifespan of the ESP.