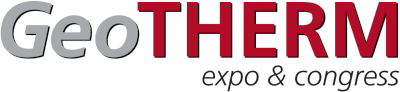
Geothermal waters still contain large amounts of thermal energy even after passing through the power plant process. Typical reinjection temperatures in the Upper Rhine Graben, for example, range from about 60°C to nearly 80°C. However, hydro-geochemical boundary conditions stand in the way of increasing the efficiency of energy production by further lowering the reinjection temperature. Highly mineralized thermal waters tend to produce more uncontrolled mineral precipitation (scaling) as the differential temperature between production and injection increases. This also applies to greater pressure relief. Scaling are a strong limiting factor for efficient and continuous operation of geothermal systems. Typically complex and site-specific thermal water chemistry complicates prediction of their occurrence. Deterministic geochemical models typically used for this purpose are strong simplifications of reality and can only ever represent a small part of the entire chemical system. Large uncertainties in the quantification of precipitates are the consequence. In the MALEG project, a new approach is being developed in which geochemical models are supplemented by artificial intelligence in order to better represent the totality of the chemical system. The AI tool will be trained with experimental hydro-geochemical data, which will be collected by power plant twins on site at the running power plant.
For this purpose, a hardware twin will be built on a demonstrator scale, which is capable of fully reproducing the processes in a geothermal plant. The demonstrator will be connected on-site, via bypass, to the geothermal plant and operated as a field laboratory for hydro-geochemical precipitation experiments and degassing processes. The operating parameters of the power plant will be run and varied up to extreme values. Close-meshed fluid and solids monitoring accompanies the experiments to evaluate possible precipitation of minerals and degassing of non-condensable gases. Changes in system parameters, such as temperature, pH, pressure, or ion concentration, provoke the formation of potential mineral phases. Experiments on at least three different geothermal systems in different geological settings and reservoirs are planned. This will create an extensive, sufficiently diverse hydro-geochemical dataset, which will form the basis for the AI prediction tool "MALEG".
MALEG's predictions are validated with a digital twin consisting of a geochemical modeling environment. The more accurate prediction of thermal water chemistry and the potential for mineral precipitation allow for a targeted optimization of operating parameters tailored to the site. These results can then be used in a further step to design an efficiency increase via cascade utilization or the integration of processes for mineral extraction, or they are the basis for planning routine monitoring programs and maintenance intervals.