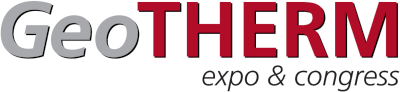
Geothermal energy is a highly reliable, eco-friendly, sustainable, and clean energy source that has proven to be a game-changer in the residential and industrial sectors. It can be developed from hot rocks saturated in geologically favorable reservoirs, in which water is produced at temperatures greater than 120 °C from a depth of up to 4 km utilizing an Electric Submersible Pump (ESP).
Due to the flow rates required, high-enthalpy fluids, and harsh downhole conditions of geothermal wells, a real-time Well Manager System was implemented to improve the ESP design, operation, reliability, and well performance. This paper details the operating conditions of a high-efficiency geothermal ESP system in Germany with in-house developed machine learning models.
Our Well Manager System has advanced to obtain virtual measurements, visual operating indices, vibrations tracking, real-time pump, and well performance evaluation, electrical unbalance tracking, and scale detection.
The machine learning models predicted pump intake pressure, motor temperature, fluid temperature, and flow rate, with less than 5% error compared to actual measurements. Additionally, the virtual parameters and real-time total dynamic head were analyzed together to indicate potential scale buildup within the flow meter or downhole components.
A thorough assessment was made by continuously monitoring (24/7/365) the physical and digital aspects of the system,
enabling recommendations to improve power consumption and increase the ESP's runlife.